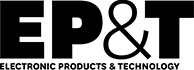
Machine learning in analog
By Tom Doyle, CEO and founder, Aspinity
Electronics Semiconductors Wireless IoT always-listening analog digital Editor Pick IoT semiconductor systemThe ultimate way to save power in IoT devices

Design engineers have tried workarounds to decrease, power consumption in an always-listening system, including duty cycling and reducing the power of each individual component.
The IoT age is upon us. Demand for more intelligent always-on connected devices is creating explosive growth in smart speakers, hearables, personal health-monitoring devices, smart home security systems, and other smart IoT products—resulting in a serious, though unintended, consequence. If IDC’s research estimate is on target, we’ll have 41.6 billion IoT devices generating an astounding 79.4 zettabytes of data by 2025, and these devices have traditionally relied on the cloud for processing. But clogged networks, as well as privacy and performance requirements, have necessitated a move to more local processing (edge processing), bringing more of the powerful cloud computing capability into the device.
It might sound attractive to move more data processing to the device edge, thereby increasing localized intelligence, but it’s not that straightforward, because as devices have become smaller and more portable, they’re also relying increasingly on battery power instead of on wall power. The recent advancements in tinyML—the integration of machine learning into small semiconductor chips—has helped to bring smart functionality into portable devices, but ultimately, the power levels are still too high for the next generation of small battery-powered devices, such as hearables and wearables, smart home-security sensing, industrial-equipment monitoring, and countless other applications.
The real challenge to power efficiency in always-on devices is the continuous collection of ambient data and its movement through the signal chain. All data are treated equally, digitized upon entry into the system, and then analyzed for a specific trigger: a wake word, a vibration anomaly, the sound of breaking glass, etc. The entire system is on all of the time just “waiting” for an event to happen. For certain devices, like those that are always listening for the sound of broken glass in a home-security system, that wait could be many months or years during which the system wastes power by digitizing and analyzing sounds that are irrelevant.
Workarounds don’t work
Tackling this power issue is also critical to keeping private data secure. Unfortunately, it’s also exceptionally difficult. Design engineers have tried workarounds to decrease power consumption in an always-listening system, including duty cycling and reducing the power of each individual component in the audio signal chain that handles the data. The reality is that these types of approaches don’t address the root cause of the problem: too much data.
To truly tackle the problem, we need to change our approach to a system solution, not a component solution. By moving to a more efficient edge architecture that intelligently minimizes the amount of data that moves through the system, we can focus the system’s energy resources on analyzing the data for a specific trigger, not on searching all sound, relevant or not.
Systems architecture is key
It’s time to move away from the digitize-first approach that has dominated the architecture of always-on edge applications since the invention of the first battery-powered edge device. In any application, we know that not all data are equally important, so if we can determine importance earlier in the signal chain, we can minimize the amount of data that moves through the full system, keeping higher-power chips in sleep mode until they’re actually needed for further analysis.
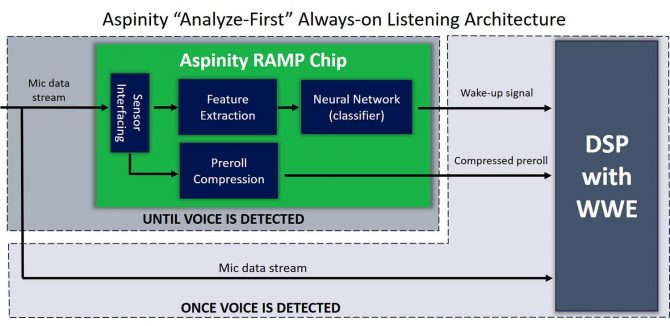
Analog machine learning (analogML) is an ultra-low power analog edge processing technology that is changing this paradigm.
For example, in a voice-first application, such as a TV remote or smart speaker, the digitize-first model requires digitizing 100% of the incoming microphone data for wake word analysis. But because voice is spoken only randomly and sporadically, up to 90% of the system power that is used to digitize and analyze the audio signal is wasted searching for a wake word when there is only noise.
Analog machine learning (AnalogML), is an ultra-low-power analog edge-processing technology that is changing this paradigm. For the first time, device designers can use analog machine learning to detect which data are important for further processing and analysis prior to data digitization. In an analyze-first architecture, the analogML chip allows the higher-power-processing components in the system, including the ADC and the DSP, to stay asleep until voice has actually been detected, and only then does it wake them to ‘listen’ for a possible wake word.
Delivering a post-microphone audio chain that draws as little as 10µA of current when always-listening, this analyze-first architecture is so efficient that it extends battery life by up to 10 times, compared to the traditional digitize-first approach to always-on listening. That’s the difference between smart earbuds that last for days, instead of hours, or a voice-activated TV remote that lasts for years, instead of months, on a single battery charge.
Ideal for portable IoT and IIoT applications (including those operating in remote locations), analogML enables the design of thousands of new types of power-efficient always-on devices that run significantly longer on battery.
————————————————
Tom Doyle, CEO & founder of Aspinity, has more than 30-years of experience in operational excellence and executive leadership in analog and mixed-signal semiconductor technology. He holds a B.S. in electrical engineering from West Virginia University and an MBA from California State University, Long Beach.